Innovation
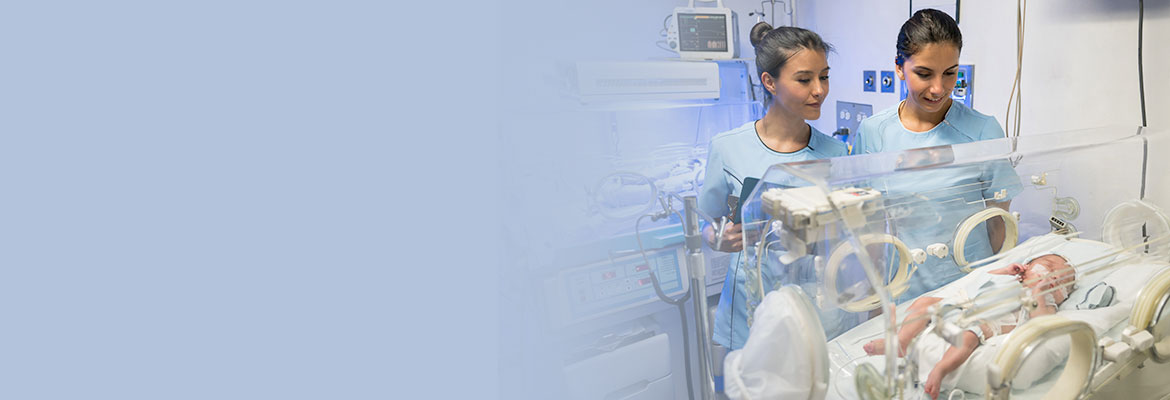
Automated Data Tool Improves Early Alerts for Sepsis
A precise and early diagnosis of sepsis is critical in premature infants, whose rates of sepsis are 200 times higher than full-term infants, to prompt rapid intervention and prevent future long-term problems such as lung disease or disability. Despite this urgency, ambiguous clinical signs and inaccuracies in screening tests can make a quick diagnosis difficult.
Researchers at Children’s Hospital of Philadelphia developed a machine-learning model that recognizes sepsis in hospitalized infants in the Neonatal Intensive Care Unit (NICU) hours before clinicians spot the life-threatening condition. In the study published in the journal PLOS One, physician-scientists and data researchers evaluated how well eight machine-learning models predicted sepsis by analyzing patient data in the electronic health record.
Because the data was retrospective, the team could compare each model’s predictions to whether or not an infant indeed developed sepsis. Six of the eight models accurately predicted sepsis up to four hours before clinical recognition of the condition. The findings are a step toward developing a real-time clinical tool that physicians can utilize in hospitals, according to first author Aaron J. Masino, PhD, an assistant professor in the Department of Anesthesiology and Critical Care and a member of the Department of Biomedical and Health Informatics at CHOP.
“Because early detection and rapid intervention are essential in cases of sepsis, machine-learning tools like this offer the potential to improve clinical outcomes in these infants,” Dr. Masino said. “Follow-up clinical studies will allow researchers to evaluate how well such systems perform in a hospital setting.”
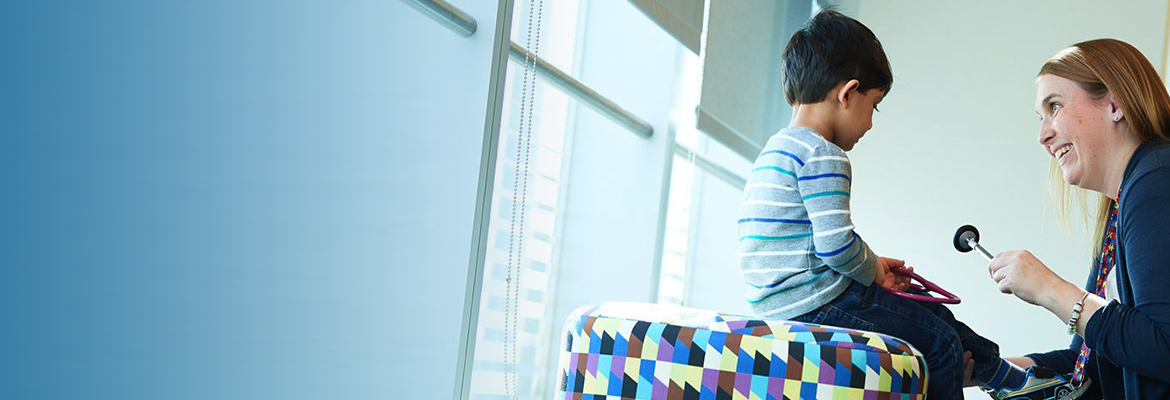
Digital Innovation Helps Quantify Autism Spectrum Disorder Assessments
With the help of a new invention from the Center for Autism Research (CAR), a simple three-minute chat can provide a powerful window of insight that can augment psychological assessment and improve doctors’ ability to measure and predict the effectiveness of interventions for complex conditions such as autism spectrum disorder (ASD). A team of scientists at the CAR Technology and Innovation Lab at Children’s Hospital of Philadelphia invented and tested the unique and fully automated method to capture, digitize, and analyze social communication between two individuals engaged in a conversation.
The portable biometric sensor system, nicknamed the Sensor Tree, is only about the size of a coffee cup, but this powerful device uses markerless motion capture technology to record and digitize all outward expressions of human behavior, including tone of voice, facial expression, motion and gestures. When combined with heart rate, temperature, and brain waves, these precision measurements can paint a highly detailed digital portrait of each person’s behavior and biology — called a “digital phenotype.”
Because social synchrony is diminished in ASD, clinician-researchers have much to gain in objectively assessing ASD by tracking behaviors like atypical eye contact or reduced nonverbal communication with exquisite precision. As machine-learning algorithms turn these granular behaviors into time-synced and objective data, the digitized method allows clinicians to move beyond a diagnostic process that, thus far, has mainly relied on human judgments.
“The Sensor Tree lets us track the coordinated facial and body movements between social partners and statistically quantifies the amount of alignment between the individuals’ movements with one another over time,” said Robert Schultz, PhD, director of CAR. “None of our current evaluations does that.”
In preliminary studies, the Sensor Tree detected with 90 percent accuracy whether a person has autism by analyzing the degree of coordination in people’s facial expressions during a three-minute conversation.
The Sensor Tree is currently being used with adults and children as young as 6 years old, and the CAR team recently received funding from the National Institutes of Health to test the device in trials with infants as an early screening tool for autism and developmental delays.
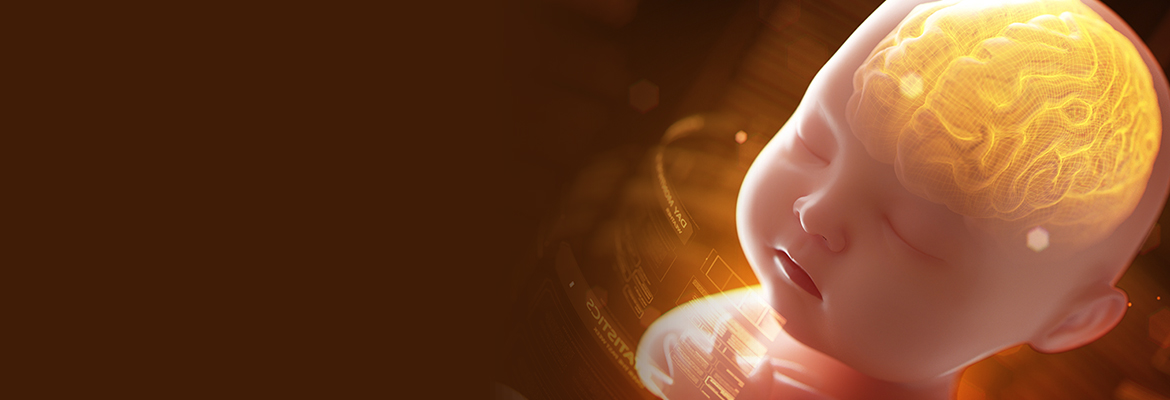
Researchers Use Cutting-Edge Imaging to Better Understand Baby Brain Development
The third trimester of life is a time of abundance and awe, as soon-to-be newborns establish complex neuronal connections in the cerebral cortex, laying down the wiring that will enable them to think, remember, and navigate the outside world. In 2019, scientists at Children’s Hospital of Philadelphia and collaborating institutions developed a novel, noninvasive method for peeking into this key window of brain development.
With immense detail, 20-minute magnetic resonance imaging (MRI) scans allow researchers to not just map how the microstructure of brain regions develops in early infancy, but identify imaging markers that will help to better understand typical and atypical brain development. The diffusion MRI scans work by detecting clues to anatomical structure based on the diffusion properties of water molecules in brain tissue, since the presence of neurons, dendrites, and other structures in the cerebral cortex are barriers to the water molecules’ movement.
“We can use quick, noninvasive brain scans as a cutting-edge way to measure microstructural maturation at critical periods of development, and then analyze those measurements in longitudinal studies that could yield new insights into the cellular processes that may go awry in neurodevelopment,” said Hao Huang, PhD, a principal investigator of Radiology Research at CHOP. “This could lead to novel screening methods that would facilitate age-appropriate interventions as soon as possible.”
Previous methods for studying the formation of neural circuits relied on time-consuming and less-detailed histological methods. Through the new method, researchers can identify the nuances of microstructural differences that may be early biomarkers for conditions such as over-connectivity, which emerges later and may be associated with autism spectrum disorder.
Publishing their findings in the Proceedings of the National Academy of Sciences, the team of researchers studied 89 preterm infants, mapping out the changes across the entire cerebral cortex. They used two types of measurements – mean kurtosis (MK) to measure microstructural complexity, and fractional anisotropy (FA) to quantify microstructural organization. Using data-driven clustering analysis of the MK and FA measurements, the researchers then identified patterns of cortical differentiation during typical brain development.
With further research underway, Dr. Huang and his colleagues plan to compile a 4-D atlas of the infant brain, which illustrates three spatial dimensions plus changes over time. Ultimately, they would like to produce a Brain Chart, or a baseline standard that outlines typical measurements of brain development much like a pediatrician’s growth chart for standard measures of height and weight.
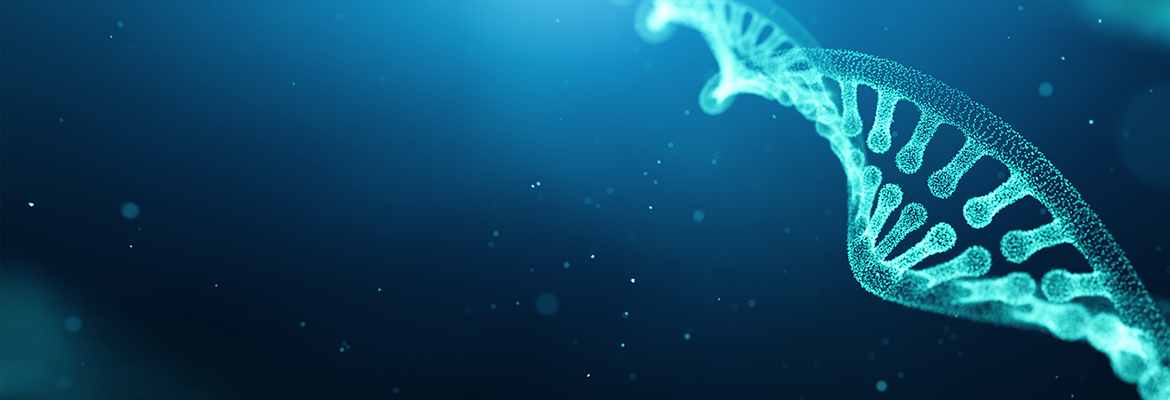
Scientists Develop Software to Streamline and Support Genetic Diagnoses
Thanks to a growing mass of genomic data, physicians and researchers discover and diagnose thousands of rare disease cases in children every day. By connecting genetic mutations in patients to hereditary conditions, they help patients and families receive answers to symptoms once deemed puzzling.
In 2019, a Children’s Hospital of Philadelphia data scientist developed a novel tool to make this process of discovery more efficient. The automated software, named EHR-Phenolyzer after its use of electronic health records (EHR), draws on information from a patient’s medical history to match potential phenotypes with gene mutations in the software’s system.
“This tool is especially relevant to a large pediatric hospital like ours, which sees many children with undiagnosed hereditary diseases,” said Kai Wang, PhD, a data scientist in the Department of Pathology and Department of Biomedical and Health Informatics who developed EHR-Phenolyzer alongside colleagues at Columbia University. “Our goal is to reduce the duration, uncertainty, and costs of the ‘diagnostic odyssey’ experienced by many affected children and their families, and to help guide them more quickly to the most appropriate clinical care.”
Dr. Wang first developed the tool’s predecessor, Phenolyzer, while at the University of Southern California. But while Phenolyzer requires clinical experts to manually input data about phenotypes, or the observable physical manifestations of a disease, EHR-Phenolyzer automates the process.
First, the software extracts clinically relevant information from the text of a narrative patient history, such as that written by a genetic counselor or clinical geneticist. The program then translates the extracted descriptors into a common language called Human Phenotype Ontology, and then matches those terms to candidate causal genes. The software prioritizes the genes by how strongly they correlate with a patient’s phenotype.
In a paper published in the American Journal of Human Genetics, Dr. Wang and his team assessed the EHR-Phenolyzer’s performance in four separate cohorts of adults and children with suspected or diagnosed genetic diseases across two centers. In more than half of the individuals, the disease-causing mutations appeared in EHR-Phenolyzer’s top 100 candidate genes; in some cases, the top 10.